Beauty of applied mathematics in research
Econometrics means the application of mathematics to the analysis of economic data. It has however raised some controversy among scholars.
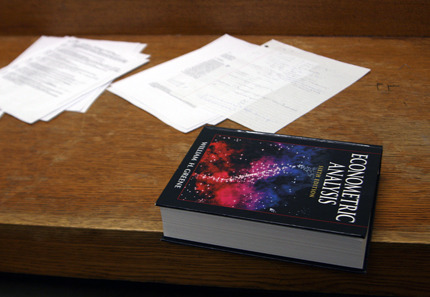
Photo: quinn.anya / Flickr.com (Creative Commons).
J. Angrist and J. Pischke in their research work present rather strong views on econometrics and its relation to the theory of economics. The central claim of the paper is the increased credibility of econometric studies in contrast to the situation thirty years ago. In fact, the article relates to an interesting assessment by E. Leamer (1983), who approached the subject matter from another point of view, namely the state of econometrics at the given time frame.
Angrist and Pischke’s presentation on econometrics attracted several statements of significance. I tend to symphatize with the opposing views as I find theoretical understanding of the underlying phenomena crucial to good experimental studies. This is because an experimental understanding without well-thought theoretical models tends to decrease our understanding of the real-world phenomena however water-proof the research design is.
Nevertheless, I believe that there is not necessarily a real disagreement between Angrist et al. and M. P. Keane, but rather minor differences on the directions of emphasis. Both authors agree that a good research design and assumptions are essential for success, while this entails a good theoretical understanding of the system under study. Most notably, to perform a good statistical analysis, it is often necessary to determine the causal relationships between the various variables. As an example, Keane notes, in closer examination the class-size results examined by Angrist and Pischke do not look very plausible.
Angrist and Pischke also refer to improved data as a source of credibility. I agree with this view in the sense that increased amount of good data tends to lower estimation variance. On the other hand, proposal on the effect of increased robustness and general understanding of linear modeling is debatable. As a matter of fact, the effect of non-linearities is hard to control. Supported by Keane’s vacuum cleaner joke (Salesman: “Ma’am, this vacuum cleaner will cut your work in half.” Customer: “Terrific! Give me two!”) non-linearities easily hinder extrapolation.
The critics mostly raise the structual approach to contrast the empirical approach promoted by Angrist and Pischke. While it’s difficult to assess the structural approach in comparison to econometrics, it does bring more theoretical understanding than quasi-experimental statistical modeling.
Keane refers to the field of marketing as a success story. While it is indeed challenging to verify, the question of dominant factors behind the developments is open. Yet, it is easy to agree with A. Nevo and M. D. Whinston on the difficulty of extrapolation and inference based on experimental studies rather than structural modeling. This is because each situation is unique.
Building statistical models that are robust and powerful enough to allow good decision-making requires strong data, as demonstrated by the merger example. On the other hand, decision-making contains factors that may be impossible to incorporate without a good theoretical model. This aspect is especially visible in macroeconomic data-analysis where data is measured over a long time period blended with major societal changes and complex underlying factors.
